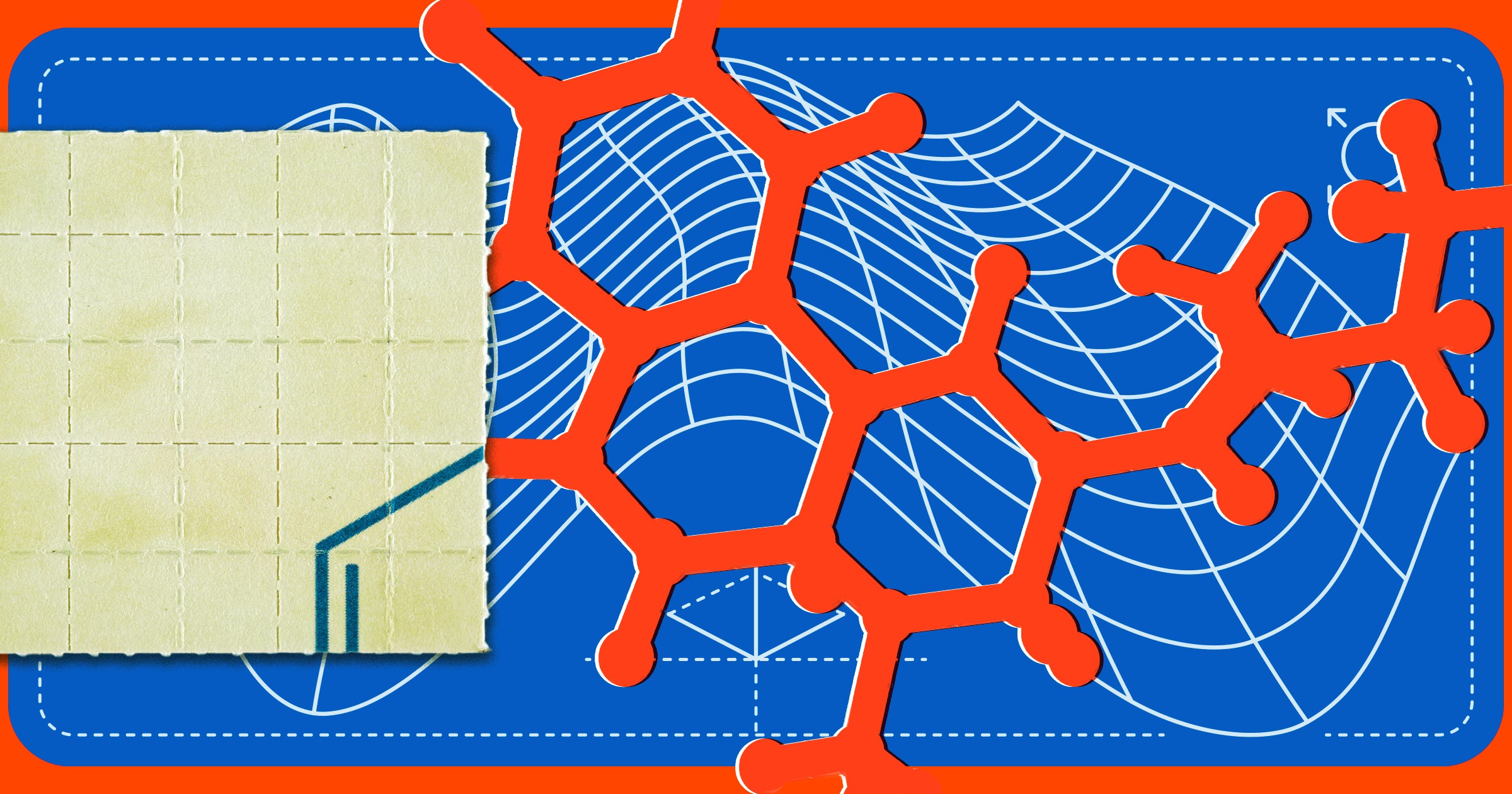
A team of researchers at the University of California, Davis, made small tweaks to the molecular structure of lysergic acid diethylamide (LSD) to see if it could be turned into an effective brain-healing treatment for patients that suffer from conditions like schizophrenia — without risking a potentially disastrous acid trip.
As detailed in a new paper published in the journal Proceedings of the National Academy of Sciences last month, the researchers created a new compound called JRT by shifting the position of just two atoms of the psychedelic's molecular structure.
With the two atoms flipped, the new drug could still stimulate brain cell growth and repair damaged neural connections, while simultaneously minimizing psychedelic effects, in mice.
"Basically, what we did here is a tire rotation," said corresponding author and UC Davis chemistry professor David Olson in a statement. "By just transposing two atoms in LSD, we significantly improved JRT’s selectivity profile and reduced its hallucinogenic potential."
In experiments involving mice, the team found that JRT improved negative symptoms of schizophrenia without worsening other behaviors associated with psychosis.
While it's still far too early to tell if JRT could be effective in humans as well, the team is hoping that the new drug could become a powerful new therapeutic, especially for those suffering from conditions like schizophrenia.
"No one really wants to give a hallucinogenic molecule like LSD to a patient with schizophrenia," said Olson. "The development of JRT emphasizes that we can use psychedelics like LSD as starting points to make better medicines."
"We may be able to create medications that can be used in patient populations where psychedelic use is precluded," he added.
Olsen and his colleagues hope their new drug could provide an alternative to drugs like clozapine, a schizophrenia treatment, without negative side effects like an inability to feel pleasure and a decline in cognitive function.
Interestingly, it also proved a powerful antidepressant in early experiments involving mice at doses 100-fold lower than ketamine, a popular anesthetic used for the treatment of depression and pain management.
But before it can be tested in humans, the team still has plenty of work to do.
"JRT has extremely high therapeutic potential," Olsen said in the statement. Right now, we are testing it in other disease models, improving its synthesis, and creating new analogs of JRT that might be even better."
More on LSD: Former CEO Sues Company That Fired Him for Microdosing LSD in an Investor Meeting
The post Scientists Tweaked LSD’s Molecular Structure and Created a Wild New Brain Drug appeared first on Futurism.
Follow this link:
Scientists Tweaked LSD’s Molecular Structure and Created a Wild New Brain Drug