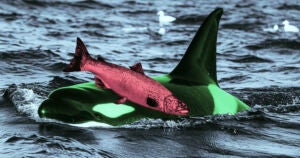
Hatful of Sorrow
You've probably heard all about orcas' daring feats, like sinking yachts. But are you aware that they can be quite dashing, too?
Yep. It appears that these snazzy cetaceans are fond of wearing "hats" in the form of dead salmon on their snouts, and sometimes other fish.
First observed in 1987, the morbid fashion trend quickly came and went like so many questionable choices in dress — but like baggy trousers, it now it appears to be back in style again.
As New Scientist reports, photographers have spotted killer whales donning salmon hats off the coast of the Pacific Northwest, reigniting debate about why orcas exhibit this puzzling behavior.
"Honestly, your guess is as good as mine," Deborah Giles, the science and research director at the non-profit Wild Orca, told New Scientist.
"We saw one with a fish on its head," she described. "So that was fun — it's been a while since I've personally seen it."
Boast to Coast
As New Scientist notes, only west coast orcas appear to favor dressing this way. East coasters, it seems, are either too cool or too démodé to bother.
Because orcas can live up to ninety years, it's possible that it's the same trendsetter from nearly four decades ago — or its acolytes — that's reviving the salmon hats. Who knew that orcas had fashion icons of their own?
"It does seem possible that some individuals that experienced [the behavior the] first time around may have started it again," Andrew Foote, an evolutionary ecologist at the University of Oslo, Norway, told New Scientist.
Fishful Thinking
In reality, there could be a practical reason behind the hats. Perhaps this is how the orcas set aside some food for later after an especially plentiful hunt.
"We've seen mammal-eating killer whales carry large chunks of food under their pectoral fin, kind of tucked in next to their body," Giles told New Scientist.
Either way, it's a testament to the intelligence of the creatures, which are social to such an almost primate degree that they develop their own cultures. It's this cultural bent — and the trends that come with it — that's believed to be responsible for how orcas not only began attacking human vessels, but learned how to immobilize and even sink them.
Nevertheless, it'll take more observations to bear out the saving-a-snack hypothesis — which Giles suggests could be done with camera-equipped drones.
"Over time, we may be able to gather enough information to show that, for instance, one carried a fish hat for 30 minutes or so, and then he ate it," she told New Scientist.
More on whales: Footage Shows Orca Blasting SeaWorld Visitors With Liquid Feces
The post Scientists Baffled by Orcas Wearing Dead Salmon as Hats appeared first on Futurism.
Read this article:
Scientists Baffled by Orcas Wearing Dead Salmon as Hats