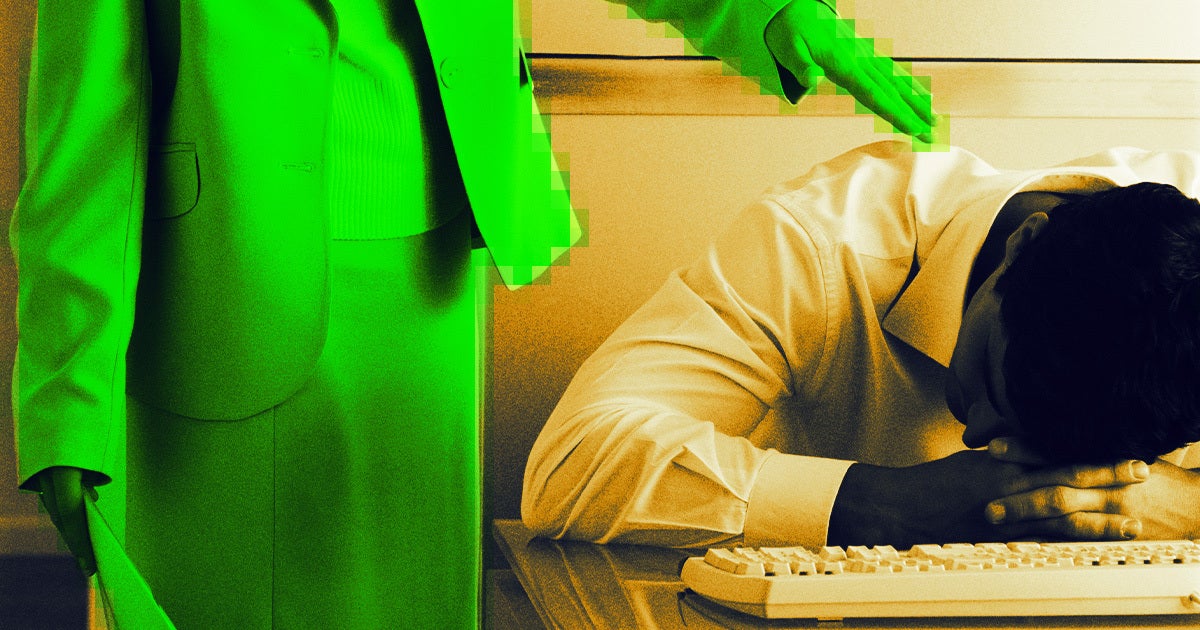
Behold Operator, OpenAI's long-awaited agentic AI model that can use your computer and browse the web for you.
It's supposed to work on your behalf, following the instructions it's given like your very own little employee. Or "your own secretary" might be more apt: OpenAI's marketing materials have focused on Operator performing tasks like booking tickets, restaurant reservations, and creating shopping lists (though the company admits it still struggles with managing calendars, a major productivity task.)
But if you think you can just walk away from the computer and let the AI do everything, think again: Operator will need to ask for confirmation before pulling the trigger on important tasks, which throws a wrench into the premise of the AI agent acting on your behalf, since the clear implication is you need to make sure it's not screwing up before allowing it any real power.
"Before finalizing any significant action, such as submitting an order or sending an email, Operator should ask for approval," reads the safety section in OpenAI's announcement.
This measure highlights the tension between keeping stringent guardrails on AI models while allowing them to freely exercise their purportedly powerful capabilities. How do you put out an AI that can do anything — without it doing anything stupid?
Right now, a limited preview of Operator is only available to subscribers of the ChatGPT Pro plan, which costs an eye-watering $200 per month.
The agentic tool uses its own AI model called Computer-Using Agent to interact with its virtual environment — as in use mouse and keyboard actions — by constantly taking screenshots of your desktop.
The screenshots are interpreted by GPT-4o's image-processing capabilities, theoretically allowing Operator to use any software it's looking at, and not just ones designed to integrate with AI.
But in practice, it doesn't sound like the seamless experience you'd hope it to be (though to be fair, it's still in its early stages). When the AI gets stuck, as it still often does, it hands control back to the user to remedy the issue. It will also stop working to ask you for your usernames and passwords, entering a "takeover mode."
It's "simply too slow," wrote one user on the ChatGPTPro subreddit in a lengthy writeup, who said they were "shocked" by its sluggish pace. "It also bugged me when Operator didn't ask for help when it clearly needed to," the user added. In reality, you may have to sit there and watch the AI painstakingly try to navigate your computer, like supervising a grandparent trying their hand at Facebook and email.
Obviously, safety measures are good. But it's worth asking just how useful this tech is going to be if it can't be trusted to work reliably without neutering it.
And if safety and privacy are important to you, then you should already be uneasy with the idea of letting an AI model run rampant on your machine, especially one that relies on constantly screenshotting your desktop.
While you can opt out of having your data being used to train the AI model, OpenAI says that it will store your chats and screenshots up to 90 days on its servers, TechCrunch reported, even if you delete them.
Because Operator can browse the web, that means it will potentially be exposed to all kinds of danger, including attacks called prompt injections that could trick the model into defying its original instructions.
More on AI: Rumors Swirl That OpenAI Is About to Reveal a "PhD-Level" Human-Tier Intelligence
The post OpenAI's Agent Has a Problem: Before It Does Anything Important, You Have to Double-Check It Hasn't Screwed Up appeared first on Futurism.